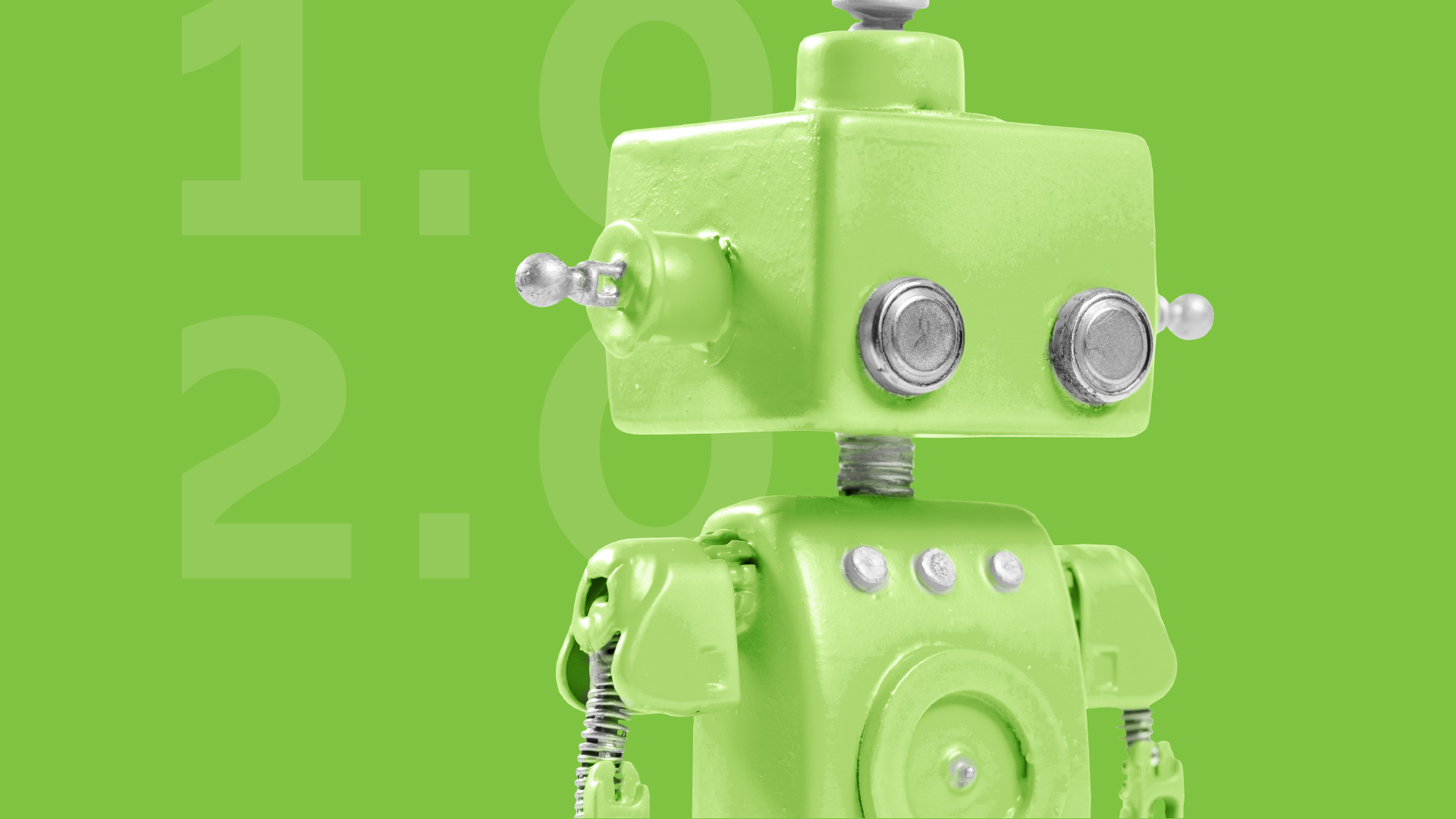
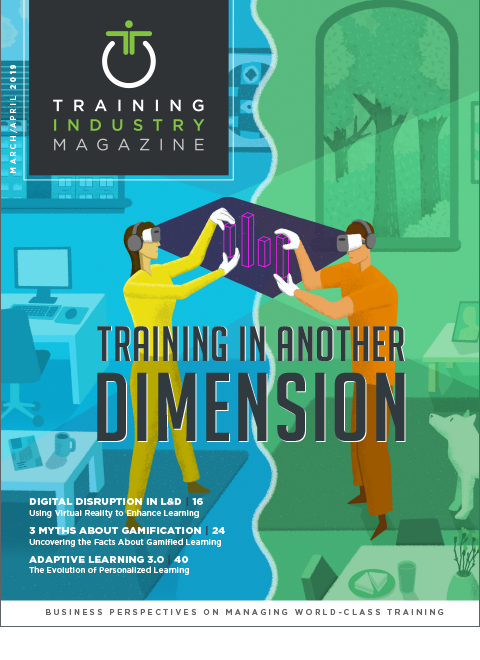
Published in Mar/Apr 2019
Adaptive learning is adapting. The adaptive learning approach, long recognized for its ability to deliver a more personalized version of learning, has a 60-year history in classrooms and corporations around the world. As technology has advanced over the decades, so has adaptive learning – becoming more refined and effective due to leaps in cloud-based managed services, computing power, scalability and machine learning.
Today, armed with great advancements in data science, artificial intelligence and machine learning, adaptive learning is on the cutting edge of a new, dramatic change.
WHAT IS ADAPTIVE LEARNING?
Adaptive learning is an instructional methodology that is designed to adjust to the needs of individual learners. Through a variety of techniques, adaptive learning technologies develop a baseline assessment of each individual’s knowledge, and then present subsequent learning that is personalized to the needs of each learner. It is characterized by:
- Intelligent adjustment of content, questions and answer choices based on performance
- Self-paced study
- Optimal, individualized learning pathways
- Immediate feedback and remediation
- An orientation toward knowledge and skill mastery
A METHODOLOGY IN FLUX
To understand how profound the next evolution of adaptive learning is, we need to start at the very beginning.
The first instance of what would become adaptive learning was conceived in the 1950s with the work of behaviorist B.F. Skinner. Skinner developed a teaching machine focused on incremental skill building. The machine adapted the questions presented based on previous correct answers, and provided students immediate feedback and the ability to move at their own pace.
Skinner’s notion of adapting learning based on individual performance progressed with the times and the technology. What we recognize as adaptive learning technology has its roots in the artificial intelligence movement in the 1970s. Researchers and practitioners began developing systems that would replicate the one-on-one instructor experience, and all the benefits therein. While the systems that resulted from this initial work were somewhat successful, computing power and AI technologies at the time simply were not advanced enough for complex intelligence or widespread use.
Adaptive learning did not die there. In fact, in the ensuing decades, adaptive learning systems became more streamlined, more scalable and more user friendly. In turn, these systems improved learning for millions of people worldwide. What began as a simplistic teaching machine blossomed into a $13 billion industry.
INTELLIGENT IN NAME ONLY
Despite the growth of the market and the advancements in adaptive systems, the prime directive of adaptive learning – to use AI to mimic one-on-one human learning – was still out of reach. AI and machine learning technology was extremely limited. Rather than giving up on the notion of adaptive learning altogether, providers devised other techniques to replicate the “intelligent experience” of AI without actually using AI.
These types of solutions typically fall into two categories:
Adaptive Learning 1.0: Basic Branching
These applications make adaptations based on decision trees and pre-diagnostics. For example, these systems might require the learner to take a pre-assessment. From this information, the system then creates a “pseudo-personalized” learning plan for the individual that focuses on their general knowledge needs. Because these plans are pre-set and heavily generalized, it means they are simply making approximations. Worse still, they are static and often become misrepresentative over time.
Adaptive Learning 2.0: Limited Algorithms
These applications make limited adaptations based on simplified algorithms. This is certainly an advancement over Adaptive 1.0, in that it automates some of the upfront manual work. It is, however, still limited and does not improve and become more intelligent over time.
While Adaptive 1.0 and Adaptive 2.0 both offer more personalization than a traditional one-size-fits-all approach, they do not really deliver on the promise of adaptive learning: a scalable, AI-driven system that mimics the interaction and learning experience of a human instructor or coach.
MAKING AI EASY
Today, dramatic advances in AI technology and the open sourcing of machine learning frameworks have enabled a very small number of elite providers to break away from the pack, and develop solutions fully powered by AI. These advancements are creating a new category of adaptive learning – Adaptive 3.0.
Adaptive Learning 3.0: AI and Machine Learning
Adaptive Learning 3.0 is characterized by the application of AI and machine learning to more accurately replicate the one-on-one instructor experience. AI-powered adaptive solutions leverage network knowledge maps to create knowledge and behavioral nodes, forming deeper relationships between content, learning objectives, and persona types, to name a few. This powers a more efficient, effective learning experience and enables:
- Complex, real-time adaptations based on learner performance and behavior
- Data-driven, personalized hints, feedback, remediation and knowledge reinforcement
- Predictive, forgetting curves and insights into future knowledge application
- Comprehensive application-level mastery of skills and knowledge
- Reduction in learning times
It is not just the learning experience that is amplified. The platforms that are fully embracing AI and machine learning are also able to provide dramatic efficiencies to learning development and content creation. For example, AI-powered platforms can assess the performance of learning content, flag underperforming content and even hide underperforming assessments until they are revised.
ADDRESSING OLD CHALLENGES
These AI-powered adaptive solutions are not just delivering on the promise of intelligent, instructor-like adaptive learning, they are also addressing some of the old weaknesses inherent in the Adaptive 1.0 and 2.0 systems. For example, AI can:
Eliminate the never-ending learning “loops”
In Adaptive 1.0 and 2.0 systems, when a learner was unable to answer an assessment correctly, the application might loop them through the same questions without any remediation or support. This “adaptive loop of death” results in learner frustration, disengagement and even distrust of the system. New AI-powered adaptive systems can provide adaptive feedback to help guide learners toward accuracy and keep them moving forward, so they do not get stuck in these frustrating loops.
Move beyond a “content first” methodology
As discussed above, some Adaptive 1.0 and 2.0 systems require learners to review all the content before getting to the meat of the learning. For learners who already have experience or knowledge on the topic, this can be a very frustrating and tedious process. As systems move toward more AI-enabled pathways, they will no longer have to insist that learners review the content before they can pass out of it. Systems will be able to ascertain, in real-time, what a learner knows and adjust the context and difficulty of the content accordingly.
Eliminate ineffective “test prep” learning strategy
Conversely, some adaptive 1.0 and 2.0 systems require learners to answer assessments upfront before they get a chance to review the content. These platforms then use learner responses to funnel each learner down a pre-set “learning pathway” wherein:
- Learners answer a series of questions
- The platform eliminates the areas of knowledge answered correctly and provides answers to incorrect assessments
- The cycle repeats until the learner answers all assessments correctly
Think of it as a technology-powered version of flashcards. The problem with this strategy is that it is designed for short-term application (like the SAT), rather than long-term corporate learning and development initiatives where ongoing application is the goal. Additionally, learners who are unfamiliar with the subject matter often find themselves in the aforementioned “adaptive loop of death.”
Adaptive 3.0 platforms deliver dynamic, real-time content adjustments based on performance and behavior, eliminating the need for upfront assessments and pre-set learning pathways. This approach is in line with the research that demonstrates the importance of self-directed learning as opposed to being funneled down a specific path. Adaptive 3.0 systems allow learners to assert control increases autonomy, which directly correlates to higher engagement, satisfaction, confidence and mastery.
Better interpret learner data
Adaptive 1.0 and 2.0 systems capture a lot of data, but often the burden of data interpretation and application is placed on the shoulders of their L&D partners. In large part, these teams are not equipped with the data science expertise necessary to make sense of vast reams of learner data. AI-powered adaptive solutions not only deliver a much stronger quality of data output – that includes follow-on predictions, recommendations and projected outcomes that mirror KPIs and strategic goals – but they also often have a data science staff on hand to help L&D partners parse insights from their data sets. Additionally, because they’re so much more robust than previous iterations, Adaptive 3.0 systems can equip learning and development leaders with measurable, quantifiable data they can take to the C-suite to inform and justify training budget spends.
Continual improvement
Finally, unlike in Adaptive 1.0 or 2.0 systems, where making updates requires intensive programming and manual coding, Adaptive 3.0 systems automatically get smarter with each user interaction. They use machine-learning algorithms to evaluate user data and continually fine-tune the platform. Additionally, adaptive systems that leverage a full feedback loop, wherein external performance data from a client can be correlated with platform learning analytics and data science, are able to unearth insights never before seen between learning and performance data.
CONCLUSION
As learning departments integrate AI-powered learning solutions, they will see a dramatic uptick in learner performance and the measurable, quantifiable business impact of their training and development programs. They will also see how AI-enabled solutions deliver more effective learning and better learner experiences – overcoming many of the traditional challenges of adaptive learning systems. The biggest benefit these learning departments will experience from Adaptive 3.0 just might be in terms of learning culture – how positively employees and workforces experience training programs and how well they buy into future opportunities for learning and training. This is the true measure of success in today’s knowledge economy and the launchpad for long-term organizational success.